23. Choose the claim about Bayesian learning that is false. (a) To compute the conditional probability P(h; | D) that the hypothesis h, is the true hypothesis to describe the world that produced the current training data D, it is necessary to first compute P(D), the probability of the set of training samples D to be observed, since this term appears as the denominator on the right hand side of the Bayes formula. (b) Once the Bayesian updating has assigned the probability of some hypothesis to be zero, that probability will always remain zero regardless of any future observations. (c) The Naive Bayes learning wields the important advantage over most other supervised learning algorithms in that some training samples can have some attributes missing without affecting the behavior of the algorithm. (d) Using only the maximum likelihood hypothesis has true error at most twice as much as the optimum Bayes classifier that computes the classification as the sum of classifications of all hypotheses h; weighted by the probabilities P(h, | D) of each hypothesis being true.
23. Choose the claim about Bayesian learning that is false. (a) To compute the conditional probability P(h; | D) that the hypothesis h, is the true hypothesis to describe the world that produced the current training data D, it is necessary to first compute P(D), the probability of the set of training samples D to be observed, since this term appears as the denominator on the right hand side of the Bayes formula. (b) Once the Bayesian updating has assigned the probability of some hypothesis to be zero, that probability will always remain zero regardless of any future observations. (c) The Naive Bayes learning wields the important advantage over most other supervised learning algorithms in that some training samples can have some attributes missing without affecting the behavior of the algorithm. (d) Using only the maximum likelihood hypothesis has true error at most twice as much as the optimum Bayes classifier that computes the classification as the sum of classifications of all hypotheses h; weighted by the probabilities P(h, | D) of each hypothesis being true.
Computer Networking: A Top-Down Approach (7th Edition)
7th Edition
ISBN:9780133594140
Author:James Kurose, Keith Ross
Publisher:James Kurose, Keith Ross
Chapter1: Computer Networks And The Internet
Section: Chapter Questions
Problem R1RQ: What is the difference between a host and an end system? List several different types of end...
Related questions
Question
Can you please help with finding one false statement below for the subject of

Transcribed Image Text:23. Choose the claim about Bayesian learning that is false.
(a) To compute the conditional probability P(h; | D) that the hypothesis h, is the true hypothesis to describe
the world that produced the current training data D, it is necessary to first compute P(D), the probability
of the set of training samples D to be observed, since this term appears as the denominator on the right
hand side of the Bayes formula.
(b) Once the Bayesian updating has assigned the probability of some hypothesis to be zero,
probability will always remain zero regardless of any future observations.
(c) The Naive Bayes learning wields the important advantage over most other supervised learning
algorithms in that some training samples can have some attributes missing without affecting the behavior
of the algorithm.
that
(d) Using only the maximum likelihood hypothesis has true error at most twice as much as the optimum
Bayes classifier that computes the classification as the sum of classifications of all hypotheses h; weighted
by the probabilities P(h; | D) of each hypothesis being true.
Expert Solution

This question has been solved!
Explore an expertly crafted, step-by-step solution for a thorough understanding of key concepts.
Step by step
Solved in 2 steps

Recommended textbooks for you
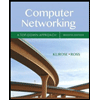
Computer Networking: A Top-Down Approach (7th Edi…
Computer Engineering
ISBN:
9780133594140
Author:
James Kurose, Keith Ross
Publisher:
PEARSON
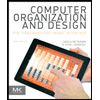
Computer Organization and Design MIPS Edition, Fi…
Computer Engineering
ISBN:
9780124077263
Author:
David A. Patterson, John L. Hennessy
Publisher:
Elsevier Science
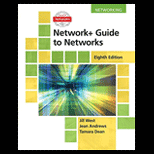
Network+ Guide to Networks (MindTap Course List)
Computer Engineering
ISBN:
9781337569330
Author:
Jill West, Tamara Dean, Jean Andrews
Publisher:
Cengage Learning
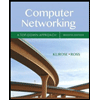
Computer Networking: A Top-Down Approach (7th Edi…
Computer Engineering
ISBN:
9780133594140
Author:
James Kurose, Keith Ross
Publisher:
PEARSON
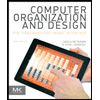
Computer Organization and Design MIPS Edition, Fi…
Computer Engineering
ISBN:
9780124077263
Author:
David A. Patterson, John L. Hennessy
Publisher:
Elsevier Science
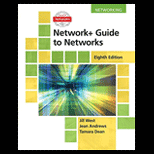
Network+ Guide to Networks (MindTap Course List)
Computer Engineering
ISBN:
9781337569330
Author:
Jill West, Tamara Dean, Jean Andrews
Publisher:
Cengage Learning
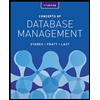
Concepts of Database Management
Computer Engineering
ISBN:
9781337093422
Author:
Joy L. Starks, Philip J. Pratt, Mary Z. Last
Publisher:
Cengage Learning
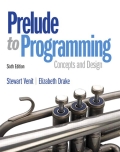
Prelude to Programming
Computer Engineering
ISBN:
9780133750423
Author:
VENIT, Stewart
Publisher:
Pearson Education
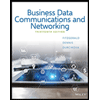
Sc Business Data Communications and Networking, T…
Computer Engineering
ISBN:
9781119368830
Author:
FITZGERALD
Publisher:
WILEY